20 May 2021
It’s 2021, yet developers writing automated tests don’t seem to be the norm to this day.
The belief that the writing of tests is just an additional effort that increases development cost is still going strong.
Of course, it’s wrong.
Yes, the learning curve is steep, and yes, there’s a lot of things to get wrong and to suffer from.
Proper developer testing like TDD is a broad topic and demands deep knowledge of design and refactoring.
None of which seem to be taught in higher technical schools that much either.
Humans have been developing software without writing tests for decades, so why bother?
Well, I write tests to my own advantage.
I do it so that I know what I’m doing, early and often.
It helps me find more joy at work and has an overall positive impact on how I feel.
Maybe also because I’m a little lazy.
Hopefully, this little diagram will help explain how this works out for me.
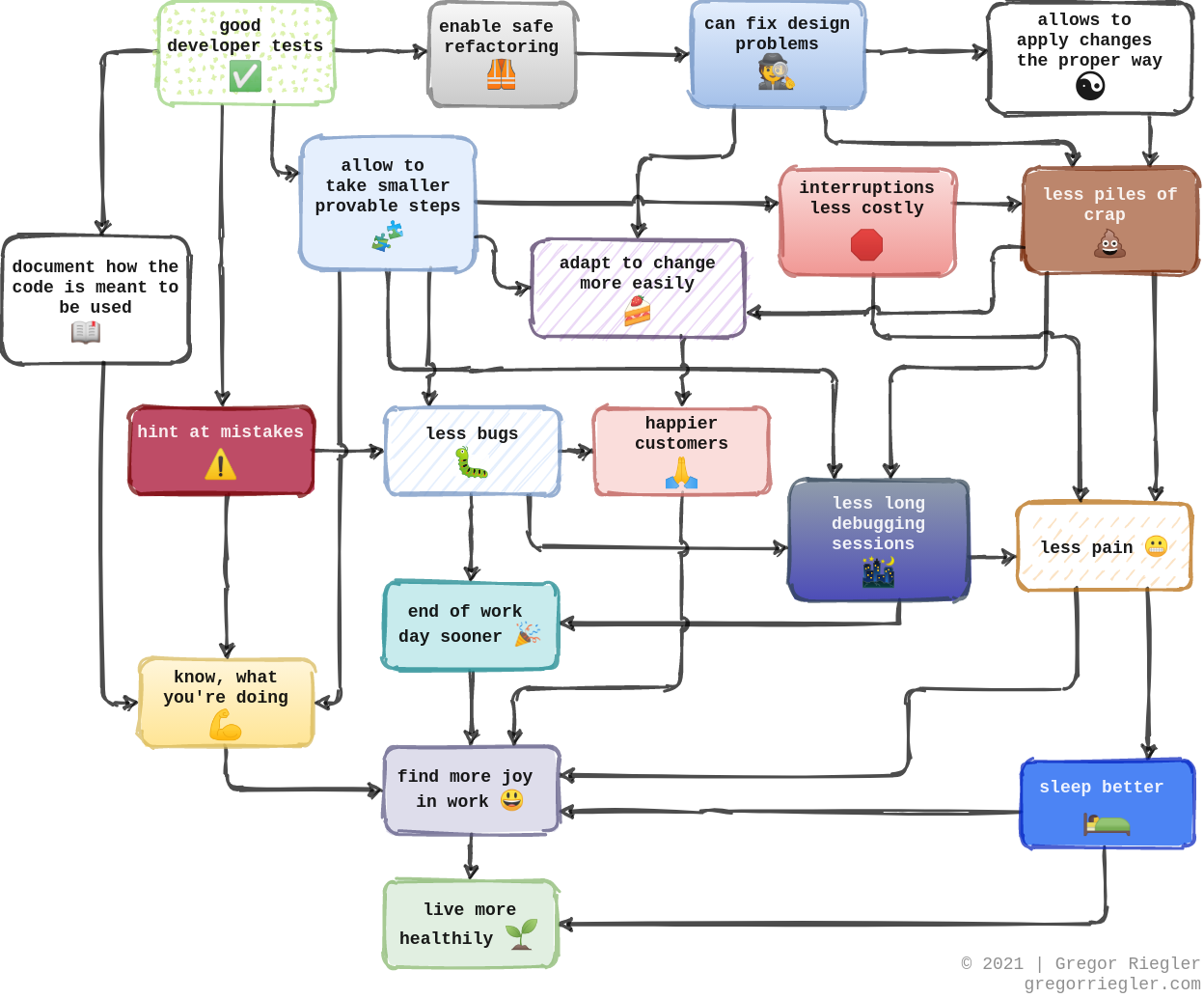
30 Nov 2020
In one of the recent Coderetreats, we did an Outside-In TDD session. I paired with a guy who was new to this, and I noticed a challenge in expressing my ideas well. Honestly, I don’t think I did a good job, so I decided to write about this topic.
A Software System
Suppose we’re developing a thing, a program.
It will inevitably become a hierarchical system composed of collaborators that form the sub- and sub-sub systems.
Each of those will solve yet another problem, and together they will form our thing.
The outer layers will be more concerned with infrastructure and coordination, whereas the inner parts will be concerned with the business logic, the domain.
Outside vs Inside
When we design the thing we can start on either side, the outside or the inside.
The Outside
The outside is where the users of the thing are.
Users might not just be humans, but also other software systems.
They observe the thing from the outside, and they cannot see inside of it.
But they don’t even care about its inside.
All they’re interested in is what it does, and how to interact with it.
So to them, it is a black box.
The Inside
The inside contains the hidden details of the thing - its gear, its structure.
It represents all the subproblems the thing was broken into.
The inside answers the question, how the thing accomplishes what it was designed for.
Inside-Out Design
In Inside-Out Design we start at the inside and gradually ascend outwards.
So we first break the problem down into smaller subproblems and define how they interact with each other.
In doing so we identify the most inner pieces, the domain of the system.
Doing Inside-Out, they are exactly where we want to start.
After all, they will be the foundation for the remainder of the system.
They are the collaborators we use as soon as we ascend to build the next higher layer.
As we ascend further and further outside we will at one point arrive at the outermost layers.
They form the interface of our system, the entry point users may interact with.
As we build those last, they will be guided by the structure and behavior of the subsystems that have already been built.
So they will be biased towards the early decisions we made when we first designed the domain.
I think that a good example of an API that is biased towards its domain is the CLI of git. You see that in the sophisticated helper tools, scripts and aliases that attempt to make it more accessible.
ⓘ Inside-Out is domain-centric. Can cause the Interface to be biased towards early domain decisions.
Stereotypes:
-
We cannot know what the domain will look like in advance, it is shaped by how users will want to use the system.
-
A bias towards the domain makes the interface more complicated.
-
To preserve a sound Interface, we might have to make ugly adjustments in the layers above the domain.
-
Thinking about usage last will cause us to build features nobody will ever use. (YAGNI)
Outside-In Design
When we start from the outside we don’t care about the domain at first.
Instead, we focus on the users and how they would want to use the thing.
So we imagine the thing as a black box while defining its interface as simple and practical as possible.
It will be doing what it should do, but we will care about that later.
Once the interface is defined we descend inwards, thinking about how we can bring this entry point to life.
Now we have to decide what collaborators will be needed, and what their responsibilities will be.
So from this perspective, the entry point we just defined is now the new user.
Again, we’re treating its collaborators as black boxes.
And again, at first, we only care about what they do, but not how.
We descend further until we arrive at the core, the domain.
As a result, the built system will be biased towards the anticipated usage of the thing.
ⓘ Outside-In is user-centric. The implemented solution might be biased towards the anticipated usage.
Stereotypes:
- We’re bad at predicting how users will want to use the system.
- A bias towards usage makes the domain unnecessarily complicated.
- Thinking about usage first will help us avoid building stuff we don’t need. (YAGNI)
Descending in Outside-In TDD
When we test drive the thing Outside-In, we may start with an acceptance test as in double loop TDD.
It describes the thing and its interface in a simple example: How it is used, and what it does.
Of course, the test does not work, as there is no thing yet.
We can now keep the test red until it passes, or we just disable it.
But our goal is to make it pass.
So we write another - this time a unit test, to guide us towards writing the code that will make the acceptance test pass.
And this is already the first of three descending strategies which I call: “Skip and Descend”.
The other two are “Fake it till you make it”, and “Replace with Test Double”.
But when we build a full slice from the entry point all the way down to the domain, we mostly don’t use just one strategy, but a combination of these.
Every time we descend we have to make another judgment call about which strategy fits best this time.
Skip and Descend
In Skip and Descend we use a test to drive the decision which immediate collaborators will be needed to suffice the test.
But we acknowledge the fact that implementing those collaborators on the basis of this test would be too big of a step.
So we disable the test and descend to start test driving the just defined collaborator.
Sometimes we may rinse and repeat until we arrive at a leaf whose unit is small enough to be implemented.
After implementing the leaf we would ascend again to the previously disabled test where we would then use the collaborator we just built.
Kind of like mikado.
Leads to sociable unit tests and test overlap.
Where test overlap happens we aim to minimize it and use the sociable unit tests to cover integrations only.
Use when
- Confident in the need of the collaborator.
- The sociable unit test will be fast:
- The collaborator is doing in-memory instructions that finish within milliseconds.
- The collaborator is going to be a fake that will be replaced by a real system later.
- The collaborator is inside the application boundary.
- The collaborator is not interacting with an expensive system such as a database or a web service.
- The call to the collaborator is not a notification.
Advantages
- Avoids test doubles, and as such decouples the tests from their implementation to enable refactoring.
Disadvantages
- Need to manage disabled tests.
- Can lead to premature collaborators.
Fake it till you make it
In fake it till you make it we don’t necessarily decide on a collaborator and descend.
Instead, we write the simplest and stupidest expression that will make the current test pass.
We then write more tests to force us to change the stupid and specific expression into something more generic.
We might have to apply preparatory refactorings in the process.
With those, we place seeds as we extract new collaborators that grow while we write and pass more tests.
May also lead to sociable unit tests and test overlap.
Use when
- Unsure which collaborators to create at first.
- The SUT (System Under Test) remains inside the application boundary.
- The SUT is not interacting with an expensive system such as a database or a web service.
- The call to the collaborator is not a notification.
Advantages
- Avoids test doubles, and as such decouples the tests from their implementation to enable refactoring.
- Collaborators emerge out of triangulation and are therefore more mature.
Disadvantages
- Testing subcollaborators from a distance.
Replace with Test Double
When we are confident in the need of a collaborator, we may decide on replacing it with a test double.
This allows us to finish the implementation of the current SUT before having to descend.
Use when
- Confident in the need of the collaborator.
- The collaborator is at the application boundary.
- The collaborator is at the boundary of the module.
- The collaborator interacts with an expensive subsystem such as a database or a web service.
- The call to the collaborator is a notification; we like to use a mock in this case.
Advantages
- Avoids test-overlap.
- Can finish the SUT before having to descend.
- Allows simulating expensive subsystems such as databases and web services.
Disadvantages
- Couples the structure of the test to the structure of the implementation.
- Mocks typically less performant than hand-written test doubles.
- Hand-written test doubles are an additional effort to write.
Conclusion
Mocks are not the only way to descend in Outside-In TDD.
There are many strategies, each of which has different trade-offs.
So we have to make a judgment call every time.
We need to keep an eye on refactorability when writing our tests.
Sociable unit tests can improve refactorability, but we have to keep the test-overlap low.
So we avoid testing all the details from a distance.
08 Aug 2020
Peter <Code Cop> Kofler and I recently facilitated a refactoring workshop where we guided our participants to incrementally extract a microservice from a monolith. Similar workshops were mostly about the technology side of things and neglected the coding part which we found was sad. So we obviously decided to focus on hands on coding and to practice the refactoring steps needed for this task.
We identified several milestones in this refactoring exercise which we then laid out as the ‘Levels of Modularity’.
Each level represents a different kind of architecture that shows stronger or weaker modularity.
The model helps us to reason about our current architecture and decide where we want to go.
But most interestingly it acts as a play book how to extract a microservice.
It is feasible to go through each level and gradually increase the modularity in an attempt to extract the microservice.
Modularity is about controlling the scope of change
Modularity is the property of a system to which degree it is composed of modules.
Modularity is recursive - Modules may be further decomposed into submodules.
A module is a cohesive, loosely coupled, encapsulated and composable piece of software that does one thing.
I don’t see modularity and its characteristics as absolutes.
I rather see nuances and trade offs.
But it is all about controlling the scope of change.
- Cohesion tells whether code that changes for the same reason, is put closely. We aim for high cohesion.
- Coupling tells to which degree a change in one part of the system affects another.
Usually we aim for loose coupling so that we can change things independently.
But the decoupling may come with a burden, which forces us to make trade offs.
So we like to find the sweet spot.
Level -1: The Distributed Monolith

Drawing |
What it means |
Grey Box with Black Border |
The Unit of Deployment |
Colored Circles |
This is Code. The Color represents what the Code is doing work for. Every Color signifies another Feature / Behaviour. |
Lines connecting Things |
Dependencies |
The structure tells that there has been an attempt to decompose the system into services.
But what it apparently does is it tears apart cohesive pieces of software and puts thick walls between them.
None of the parts is able to perform useful work on its own. They have a strong coupling with their dependencies.
Shotgun Surgery is the result.
One has to touch many services when changing a single feature.
Another effect is that it’s mostly impossible to deploy any of those services independently.
I put this even below level 0, as i see negative modularity going on.
In an attempt to increase its modularity we would first have to join the services again, only to arrive at level 0.
How did we get there?
- We just didn’t understand how to properly decompose a system into modules.
- We drew the boundaries before we had a proper understanding of the domain we’re building.
- There are different developers working on different layers of the same thing. (Conways Law)
Level 0
Monoliths with low cohesion and high coupling.
Level 0: The Big Ball of Mud

This animal is completely disorganized. There is no recognizable structure, no abstraction, dependencies are intertwined.
Strong coupling is the result. Changes in one area of the code lead to bugs in several other areas.
It contains all sorts of Code Smells from God Classes to Feature Envy, Dead Code and Change Preventers.
Developers get there because of many reasons:
- Lack of Experience
- Carelessness after feeling treated unfair
- Rushing Changes to try and meet a deadline
- Developers with different views working independently on the same codebase without sufficient alignment.
- Fluctuation
Level 0: Monolith packaged by Layer

This is probably the most common monolith out there.
Its package structure is often guided by frameworks and code generators that propose having packages like controllers
, domain
and repositories
on the top level.
This framing proposes Separation of Concerns which is a good thing.
But the proposed way of having those packages on the top level obfuscates what the application is doing. “Controllers” does not provide any clue about what the app is doing.
Level 1
Monoliths with high cohesion.
Level 1: Package by Feature

Package by Feature is much more desirable.
It puts the names of features like Order, Cart and User at the top level which are screaming: “I am a Shop!”.
It helps us to quickly find the code we need to change.
As the cohesion is increased, we typically just have to touch a single top level package when changing a feature.
There is no information hiding going on between the features though.
A code within one feature knows about the internals of another feature and has access to it.
For Example: Feature A may fetch data from the Repository of Feature B, or use its business logic.
So there is still coupling going on.
Level 1: Features, but layered inside

Separation of Concerns again, just inside feature packages.
We do this when we want to isolate our domain, our Happy Zone from infrastructure code.
Or maybe we just like to organize our many files and put them into distinct folders to gain a better understanding of what a file is about.
Level 2: The Modular Monolith

A Monolith with both low coupling and high cohesion.
Feature packages turn modules.
Each module now has a clear api which is the only way to access it.
One module doesn’t know about the inner details of another module anymore.
So they are encapsulated, and the coupling is further reduced.
But as the module does not encapsulate its data, the modules are still coupled by the shared database.
Increasing the modularity further than this may come with a significant cost.
Level 3: The Microservice Ready Monolith

The coupling of a shared database is removed.
Each module now uses its own data.
Could be a separate database, or just separate tables within the same database.
As long as there is no hidden coupling like a foreign key, we consider it as separated.
Sometimes, two or more modules need to access the same data.
In this case they would access the data through the module that owns it, or have a separate copy of the data.
In case of a copy we would choose a data model that perfectly fits the needs of the respective module.
The data might be updated through events.
It’s still a monolith, but a very decoupled one.
Extracting a microservice is very easy from this level.
Level 4: Microservices

We can now deploy, as well as scale each service independently.
Each microservice has a strong autonomy and can be developed by its own team that uses the tech that best fits the services job.
But it comes at a cost and a lot of pain:
- Hard to Integrate.
- Hard to Maintain.
- An organizational challenge.
- Lot’s of additional Technology might be needed.
- API Gateway
- Event Bus
- Container Orchestration
- Configuration Broker
- Contract Testing
- Bulkheads
- Circuit Breakers
- Distributed Tracing
- Distributed Logging
- Service Registry
- and so on
“Don’t even consider microservices unless you have a system that’s too complex to manage as a monolith.”
~Martin Fowler
Conclusion
The Levels of Modularity provide a model to reason about an architecture and help decide on where you want to go.
The higher the level, the more modular an architecture is.
Also, we may use the Levels of Modularity as a cook book to refactor to a more modular architecture.
We want to go level by level incrementally.
To get from a Big Ball of Mud (Level 0) to a Modular Monolith (Level 2), we first want to find the features and collect them in distinct packages to arrive at Package by Feature (Level 1).
Only then would we decouple the feature packages to finally arrive at a Modular Monolith (Level 2).
We may even apply the Strangler Pattern and carry one feature at a time through all levels.
11 May 2020
Consumer Driven Contracts
Consumer Driven Contracts (CDC) is a pattern that takes Outside-In TDD across boundaries, and is frequently used in Micro Service Architectures.
Note that TDD is not primarily a testing process, but a design technique.
The same is true for Consumer Driven Contracts where Consumers drive the capabilities of Producers by expressing a clear need.
This ties service evolution to business value.
Consumers start by writing Contract Tests and define what a Producer’s API should be like.
The Producer is replaced by a Mock Object whose configuration generates the Contract.
The interactions described in the Contract are later replayed against the Producer in a test harness.
Both, the Consumer and the Producer are tested in isolation and evolve independently.
Driving Client Code Via Consumer Tests
Another thing that the Consumer’s Contract Tests are good for is they can help emerge the client code.
We aim for a client code that hides the technical details such as HTTP messaging and JSON serialization from us.
The client code should represent the remote service within our boundary and hide the fact that it is remote.
Defining A First Contract
We’re building a Book Store, so we need a Book Catalogue as a Producer.
It should serve the Book’s information.
Let’s start with a happy path for finding a single Book.
This is what the Producer Mock would look like using Pact JS.
The definition of this Mock as well generates the Contract.
I’m skipping the boilerplate, you can look it up in my github.
const requestASingleBook = {
state: "two books",
uponReceiving: "a request for retrieving the first book",
withRequest: {
method: "GET",
path: "/books/1"
},
willRespondWith: {
status: 200,
headers: {"Content-Type": "application/json"},
body: {
"self": "/books/1",
"title": "Title"
}
}
}
Choices, Choices, Choices
We can now start writing the actual Consumer Test.
But where do we start?
What do we assert?
We could try to design the client code by wishful thinking.
it("finds a single book", () => {
// represents the producer
const books = new Books()
// executes the http call and does json deserialization
const book = books.byId(bookId)
expect(book).to.deeply.eq(expectedBook)
})
This could be a first failing test, and a nice design that hides the technical details as desired.
But it certainly is a bit premature.
We haven’t even put an API’s baseUrl.
Neither did we think about potential errors.
All the HTTP handling code would have to go to the not yet existing Books
object.
How would we even go about making a HTTP request?
How would we deserialize the content?
There are many things coming up, and it already feels like a huge leap.
There has to be a better way.
Making Smaller Steps
We could just ignore the design for now and focus on the technical details.
Meaning we would start and implement the HTTP call within the test.
This approach puts us closer to the technical details.
It allows us to fiddle around, and prevents us from designing prematurely.
Later, when we experience duplication, we can extract the implementation code from the test to emerge a more mature design.
Some would call this TDD as if you meant it (TDD aiymi).
A Failing Test
We start by defining our final expectation, that is the book data as a result.
We don’t yet know how to get there.
All we know is the url.
Note how we don’t assert on any technical details like the HTTP Status Code and Content-Type.
These details don’t concern us, they will be hidden once we’re finished.
const requestASingleBook = {
state: "two books",
uponReceiving: "a request for retrieving the first book",
withRequest: {
method: "GET",
path: "/books/1"
},
willRespondWith: {
status: 200,
headers: {"Content-Type": "application/json"},
body: {
"self": "/books/1",
"title": "Title"
}
}
}
// ... boilerplate ...
it("finds a single book", async () => {
const url = "http://localhost:1234/books/1"
const result // ??
expect(result).to.deep.eq({self: "/books/1", title: "Title"})
})
Run the tests => Red.
Make it Green
Let’s find the simplest way to make the test green by writing all implementation code inside the test.
it("finds a single book", async () => {
const bent = require("bent") // bent is just a http client
const getJSON = bent("json")
const result = await getJSON("http://localhost:1234/books/1")
expect(result).to.deep.eq({self: "/books/1", title: "Title"})
})
Run the tests => Green.
Fabulous!
Refactor
As it works we can now think of improving it.
Let’s create a type for the book.
it("finds a single book", async () => {
const bent = require("bent")
const getJSON = bent("json")
type Book = {
self: string
title: string
}
const result: Book = await getJSON("http://localhost:1234/books/1")
expect(result).to.deep.eq({self: "/books/1", title: "Title"})
})
Run the tests => Green.
Sticking To The Robustness Principle
⚠ The Robustness Principle says that we should be conservative in sending stuff but liberal in receiving it.
The goal of this is to reduce the risk for messages to fail.
Consumers should be tolerant to API change, able to survive most of it.
They should only be concerned with the Resources, Methods and Fields they actually consume, and treat even them with care.
In the existing solution a new arriving field would cause the message to fail.
Let’s imagine the server would add a new field: description
.
Our client code would not expect it and break.
We need to make it more robust and prevent this from happening.
But, should we not write a test first proving that it would break?
Totally!
However, we are currently in the writing of a Consumer Test.
It generates the Contract for the Producer.
Adding the description
field to the Contract is not what we want.
We only want to add the fields that we consume.
So let’s
- add the field temporarily to prove that it breaks,
- fix the implementation to not break anymore,
- and finally remove the field again.
it("finds a single book", async () => {
const bent = require("bent")
const getJSON = bent("json")
type Book = {
self: string
title: string
}
const decodeBook = (object): Book => ({
self: object.self,
title: object.title
});
const response = await getJSON("http://localhost:1234/books/1")
const book: Book = decodeBook(response)
expect(book).to.deep.eq({self: "/books/1", title: "Title"})
})
Run the tests => Green.
The decodeBook
function makes the code robust against any change to fields that we don’t consume.
Aiming For Duplication
Already fiddling around a lot, aren’t we?
Let’s aim for some duplication by implementing the not found case.
We can just copy and paste the earlier test and adapt it.
Shouldn’t have to change much, should we?
const requestANonExistingBook = {
state: "two books",
uponReceiving: "a request for a non existing book",
withRequest: {
method: "GET",
path: "/books/3"
},
willRespondWith: {
status: 404
}
}
// ... boilerplate ...
it("finds nothing", async () => {
import {none, Option} from "fp-ts/lib/Option"
const bent = require("bent")
type Book = {
self: string
title: string
}
const decodeBook = (object): Book => ({
self: object.self,
title: object.title
});
const getStream = bent("http://localhost:1234/books/3", 200, 404)
const stream = await getStream()
let book:Option<Book>
if (stream.status !== 200) {
log("received " + stream.status + " " + await stream.text())
book = none
}
expect(book).to.equal(none)
})
Run the tests => Green.
That escalated quickly.
The implementation changed quite a bit.
We had to change the way we use bent to support the 404 status code.
Also, we decided to introduce the Option type to make explicit a book might not be returned.
The two tests look quite different now.
Finding The Common Denominator
Before we can extract it as production code we need to adapt both tests to match each other.
it("finds a single book", async () => {
import {none, Option, some} from "fp-ts/lib/Option"
const bent = require("bent")
type Book = {
self: string
title: string
}
const decodeBook = (object): Book => ({
self: object.self,
title: object.title
});
const getStream = bent("http://localhost:1234", 200, 404)
const stream = await getStream("/books/1")
let book:Option<Book>
if (stream.status !== 200) {
log("received " + stream.status + " " + await stream.text())
book = none
} else {
book = some(decodeBook(await stream.json()))
}
expect(book).to.deep.eq(some({self: "/books/1", title: "Title"}))
})
// ...
it("finds nothing", async () => {
import {none, Option, some} from "fp-ts/lib/Option"
const bent = require("bent")
type Book = {
self: string
title: string
}
const decodeBook = (object): Book => ({
self: object.self,
title: object.title
});
const getStream = bent("http://localhost:1234", 200, 404)
const stream = await getStream("/books/3")
let book:Option<Book>
if (stream.status !== 200) {
log("received " + stream.status + " " + await stream.text())
book = none
} else {
book = some(decodeBook(await stream.json()))
}
expect(book).to.equal(none)
})
Run the tests => Green.
Ok, great.
The implementation code is now the same in both cases: Finding a book, and not finding it.
We can finally start and extract the duplicated parts out of the tests.
Let’s begin with the Book type, and the decodeBook
function.
import {none, Option, some} from "fp-ts/lib/Option"
const bent = require("bent")
type Book = {
self: string
title: string
}
const decodeBook = (object): Book => ({
self: object.self,
title: object.title
});
// ...
it("finds a single book", async () => {
const baseUrl = "http://localhost:1234"
const path = "/books/1"
const getStream = bent(baseUrl, 200, 404)
const stream = await getStream(path)
let book:Option<Book>
if (stream.status !== 200) {
log("received " + stream.status + " " + await stream.text())
book = none
} else {
book = some(decodeBook(await stream.json()))
}
expect(book).to.deep.eq(some({self: "/books/1", title: "Title"}))
})
// ...
it("finds nothing", async () => {
const baseUrl = "http://localhost:1234"
const path = "/books/3"
const getStream = bent(baseUrl, 200, 404)
const stream = await getStream(path)
let book:Option<Book>
if (stream.status !== 200) {
log("received " + stream.status + " " + await stream.text())
book = none
} else {
book = some(decodeBook(await stream.json()))
}
expect(book).to.equal(none)
})
Run the tests => Green.
Note how I as well extracted both the baseUrl
and path
variables inside the tests.
This allows us to further extract the function that does the actual request.
import {none, Option, some} from "fp-ts/lib/Option"
const bent = require("bent")
type Book = {
self: string
title: string
}
const decodeBook = (object): Book => ({
self: object.self,
title: object.title
});
async function findBook(baseUrl: string, path: string): Promise<Option<Book>> {
const getStream = bent(baseUrl, 200, 404)
const stream = await getStream(path)
let book: Option<Book>
if (stream.status !== 200) {
log("received " + stream.status + " " + await stream.text())
book = none
} else {
book = some(decodeBook(await stream.json()))
}
return book
}
// ...
it("finds a single book", async () => {
const baseUrl = "http://localhost:1234"
const path = "/books/1"
const book = await findBook(baseUrl, path)
expect(book).to.deep.eq(some({self: "/books/1", title: "Title"}))
})
// ...
it("finds nothing", async () => {
const baseUrl = "http://localhost:1234"
const path = "/books/3"
const book = await findBook(baseUrl, path)
expect(book).to.equal(none)
})
Run the tests => Green.
Final Touch
The tests became nice and short.
However, I would prefer having a bookClient object that had the baseUrl
baked in.
This would allow me to add more functions later.
So I refactor it further, and extract the module.
The final result looks like this:
// content of book-client.ts
import {none, Option, some} from "fp-ts/lib/Option"
const bent = require("bent")
export function createBookClient(baseUrl: string) {
type Book = {
self: string
title: string
}
const decodeBook = (object): Book => ({
self: object.self,
title: object.title
});
return {
requestBook: async (path: string): Promise<Option<Book>> => {
const getStream = bent(baseUrl, 200, 404)
const stream = await getStream(path)
if (stream.status !== 200) {
log("received " + stream.status + " " + await stream.text())
return none
}
return some(decodeBook(await stream.json()))
}
}
}
// content of tests
import {createBookClient} from "./book-client"
// ...
const client = createBookClient("http://localhost:1234")
it("finds a single book", async () => {
const book = await client.requestBook("/books/1")
expect(book).to.deep.eq(some({self: "/books/1", title: "Title"}))
})
// ...
it("finds nothing", async () => {
const book = await client.requestBook("/books/3")
expect(book).to.equal(none)
})
Run the tests => Green.
And we are finished.
Conclusion
We drove a clean client code from writing Consumer Tests.
We used the tests to fiddle around with technical implementation detail.
Once we had tested more cases, we aligned their implementations to extract common code.
The design we arrived at is a little different from the one we had originally anticipated.
TDD leaves us with a choice when to design.
We can do it upfront by wishful thinking - in the Red Phase.
Or we can defer it to a later stage - in the Refactoring Phase.
The latter makes it easier to fiddle around with the details and may lead to a more mature design.
You can find the code on my github.